ORIP-Supported Supercomputers Help Advance Radiomics
The rapid expansion of artificial intelligence (AI) technologies in recent years holds considerable promise for biomedical research and the advancement of techniques that can improve patients’ lives. One of these techniques is radiomics, which involves the computerized analysis of features from many medical images in the development of AI algorithms that can identify tumors and other abnormalities. “Radiomics is utilizing machine learning and AI methods to interpret and extract information from images that are complex and not visually perceptible, and we can learn a lot more than we actually see using conventional methods,” explained Dr. Christos Davatzikos, Wallace T. Miller Senior Professor of Radiology, Informatics and Electrical and Systems Engineering and Director of the AI2D Center for AI and Data Science for Integrated Diagnostics at the University of Pennsylvania. Advanced computing power is key to radiomics techniques. ORIP has supported several supercomputers used for radiomics through its S10 Shared Instrumentation Programs, especially the High-End Instrument Program, including instruments at The University of Chicago (S10RR021039, S10OD025081) and the University of Pennsylvania (S10OD023495).
Dr. Maryellen Giger, A.N. Pritzker Distinguished Service Professor of Radiology at The University of Chicago, has been developing radiomics techniques since the 1980s to interpret images of potential cancers and define characteristic elements to aid in providing a diagnosis. “We’re interested in finding disease early,” Dr. Giger commented. “The earlier we find it and diagnose it correctly, the better the cure.” Radiomics is a noninvasive technology that analyzes standard medical images to develop a more thorough understanding of disease characteristics, which can lead to earlier diagnosis of cancers and other potential issues. The University of Chicago has received two S10 grants for high-performance computing systems that use deep-learning techniques to analyze and interpret medical images (Figure 1). “With radiomics,” Dr. Giger explained, “we’re using image processing and image analysis techniques to extract characteristics of abnormal and normal areas, come up with mathematical relationships for predictive models, and convert them to software, for example, to characterize the fuzziness of a tumor margin in predicting the likelihood of malignancy.”
At the University of Pennsylvania, Dr. Davatzikos and his team use their ORIP-supported supercomputer (Figure 2) to study brain cancer, neurodegenerative diseases, and neuropsychiatric disorders. “By looking at radiomic features and incorporating them into machine-learning predictive models, we can actually predict, to some extent, patient survival. We can also predict where the tumor is infiltrating beyond the visible margins,” Dr. Davatzikos explained. His team also has been developing ways to identify more subtle phenotypes of aging and other neuropsychiatric issues that normally are not visible on brain scans. The technology used in radiomics has become advanced enough to detect patterns of subtle early brain changes that could be predictive of later progression to dementia or other serious disorders.
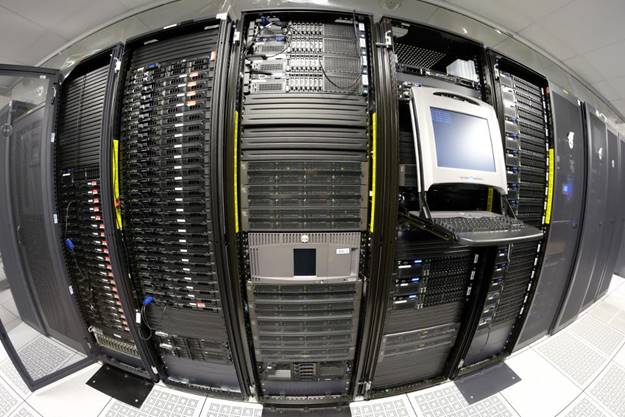
Radiomics requires large amounts of data and significant computing power to identify these characteristic features of diseases and conditions. Using high-powered computers enables Dr. Giger, Dr. Davatzikos, and their teams to analyze images and data from many patients and better identify patterns that may correlate to specific phenotypes or stages of disease. To develop more accurate results, larger amounts of data are needed. One challenge is that many existing data sets are less diverse than the general population, so results that are accurate for one group of people could be inaccurate for a patient with a different genetic or demographic background. “We’re in the era of big data,” Dr. Davatzikos said. “The more diverse the data set is, the better you learn these radiomic patterns.”
Dr. Giger emphasized that, despite the availability of faster computers, larger data sets, and more advanced algorithms across many different disease sites and modalities, substantial development in the field of radiomics is still needed to ensure the repeatability and reproducibility of the information that is derived. With many types of diseases, not enough data sets are available to train a radiomics algorithm to identify disease-relevant features. The recent movement to improve data sharing in biomedical research will help improve radiomics by making data broader and richer. “We need to convince people more and more to open their data,” Dr. Giger said, emphasizing the need to continue the movement toward open science and to change the culture to increase knowledge of AI techniques among study sections.
Dr. Davatzikos agreed that data standardization and harmonization are critical for ensuring that the algorithms developed from these data are as predictive as possible. “Most people who work on AI, they now acknowledge that it’s only in the data. The data will determine how good your algorithm is,” Dr. Davatzikos said. “Having good quality and diverse, rich data that capture all aspects of the phenomenon that you’re trying to capture is very important.” Technology advances such as deep learning have brought the promise of precision and personalized medicine closer than ever, but algorithms built from incomplete and biased data cannot provide accurate results to all people. Dr. Davatzikos pointed out that the increasing ability to see subtleties in the data will help researchers better define a greater number of conditions, allowing them to investigate the mechanisms of each and develop better treatments for each individual patient.
One of the next areas of progress for the field of radiomics is increasing the ability to make connections across disciplines and diseases. Radiomics is inherently multidisciplinary—requiring the expertise of clinicians, imaging scientists, engineers, biomedical researchers, and computer scientists—but current radiomics research is focusing on discrete topics and more work is needed to be able to apply the relevant techniques in multiple fields at once. “We can demonstrate that there are methods translated in breast cancer, in thyroid cancer, in heart disease. But ultimately, you would like to submit the chest X-ray and look at it and say, ‘Okay, we’ve covered these 20 diseases,’” Dr. Giger said. She suggested that the ideal would be to have radiomics algorithms that could mimic every test on a physician’s report, providing thorough and efficient exams with maximal benefit to the patient.
A larger challenge for the field is the need to increase acceptance of AI techniques to better integrate radiomics into clinical practice. Dr. Giger stressed that AI functions complement, rather than replace, the actions of human clinicians when assessing medical results—radiomics techniques can suggest whether a tumor is likely to be malignant or benign, but a human will use that information as only part of their medical decision-making. “It’s like finding items with red and white stripes” on a “Where’s Waldo?” picture, Dr. Giger explained. “A human can make the decision that ‘that’s a Waldo, and that’s not a Waldo.’” Using algorithms to help with decision-making in the clinic has increased acceptance of the technologies, but the increasing popularity of AI in general also can generate the perception that the technology is more advanced than it is. “There’s a bit of a gap between the state of the science and what is being used in the clinic, and that is difficult to bridge,” Dr. Davatzikos pointed out.
“The field is evolving very fast,” Dr. Davatzikos said. “You want to build on something, but you know the next year something is going to change, so you’re at risk of chasing your tail all the time.” Although the ORIP-supported instruments were critical to developing radiomics research at The University of Chicago and the University of Pennsylvania, computational needs that are required to keep up with deep-learning technologies are growing exponentially. This situation highlights the challenge of meeting the growing need for faster, more powerful state-of-the-art computational infrastructures. The field of radiomics holds great promise for patients and for personalized medicine; ensuring that researchers can access the latest technologies is crucial to moving the field forward. “I think that in the next 10 years, we will see a transformation,” Dr. Davatzikos said, predicting the widespread adoption of AI and radiomics techniques. “These changes are like phase transitions in chemistry and physics, where all of a sudden the thinking flips and everybody starts to use them.” ORIP’s support is critical to driving these changes and allowing the field to flourish. “We’re grateful to NIH,” Dr. Giger said. “We could not have succeeded in our research without them.”
ORIP’s S10 programs support purchases of state-of-the-art, commercially available instruments to enhance research of NIH-funded investigators. S10 awards are made to domestic public and private institutions of higher education, as well as nonprofit domestic institutions, such as hospitals, health professional schools, and research organizations. Every instrument awarded by an S10 grant is to be used on a shared basis, which makes the programs cost efficient and beneficial to thousands of investigators in hundreds of institutions nationwide. For more information, please visit ORIP’s S10 Instrumentation Programs webpages.